In 2021, Pave was on its way to build the largest real-time dataset for compensation data. At the time, we had 1,000+ companies within our dataset, 100K+ data points, and countless sources to supplement our findings.
We initially followed the industry standard to build our equity benchmarks, but realized something was missing. The results were all over the place and conflating multiple forces that might drive equity value.
So what was the industry getting wrong?
Let’s dive a bit deeper into the problem.
The State of Equity Data
Founders, people leaders, VCs, and anyone else responsible for equity compensation have always lamented the lack of reliable equity data. Reports on equity benchmarks only share the “current value” of employee equity, big numbers that are more reflective of rising valuations in a hot funding environment over the past decade.
But these numbers don’t tell the story of what those employees were granted when hired. A natural limitation to prior survey-based approaches for benchmarking is the ability to only show users as a snapshot in time, without historical context.
One of our customers, Harshita Arora, Co-Founder of AtoB, shared her experience about this:
“Hiring top talent has never been harder, so it’s critical that AtoB is staying ahead of compensation trends in the job market. Equity is a critical component of the offers we make and, until now, it’s been impossible to find reliable and up-to-date equity benchmarks for new hires. This new offering from Pave will help us move faster and make better equity-based compensation decisions.”
I can get a sense of the amount a software engineer is vesting after their company 20x’d in the last two years, but this doesn’t tell me what they were granted when joining the company. This new offering from Pave will help us make better equity-based compensation decisions.”
Stories like these tell us that there are three problems at work.
First, companies aren’t looking at the full picture for equity benchmarking.
Second, there is a lack of trust around equity, compensation, benchmarks and salary. The data reflects a snapshot in time, without much context.
And third, companies are struggling to recruit talent because they don’t know what makes for a fair and competitive offer.
Imagine an engineering leader trying to grow her team at a Series B company. When she logs into a traditional survey tool, she will see a massive range of equity benchmarks driven primarily by non-uniform company growth in valuations. At best, she will have to use a rule of thumb to adjust the equity data to normalize benchmarks to what they would have been at the time those employees were hired.
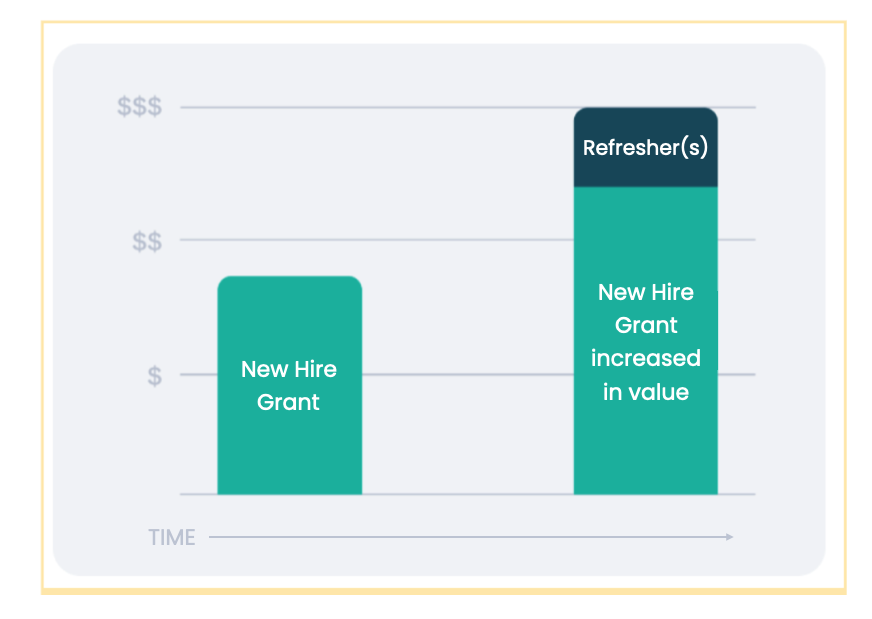
As a result, executives are forced to make guesses at what new hires should get from an equity perspective. As you can imagine, that process is filled with bias and can cause inequity down the line.
Even worse, those inequities are really hard to fix. Where cash inequities can be fixed in a single merit cycle, it’s hard to get people back on equal footing after grants are given. Over time, equity value grows and the gap gets bigger and bigger. Messing up the equity conversation from the start is a one way door to an unequal culture.
Equity compensation is an arguably larger component of total compensation, and realized that we had to fix this.
The Solution: Pave Launches New Hire Equity
We pride ourselves on being a comptech leader, but under the hood we’re closer to a data integrations company. Our tool supports a web of complex and critical interfaces that allows our product to surface insights from our thousands of customers.
To solve this problem, we expanded our integrations, surfacing key information about equity grants – like date granted, value, and any additional grants awarded – that make it possible to distinguish total equity, the legacy approach, from the new hire equity value.
This novel dataset combined with the scale of our customer base, produced a first-of-its kind product to give users the context to make more educated decisions for equity benchmarking.
Let’s take a quick look at the new feature!
How New Hire Equity Helps People Leaders
With the launch of this new product, companies have access to a previously unavailable dataset that allows them to more easily and confidently create offers involving new hire grants. It delivers accurate and comprehensive equity data for new hires across a range of roles, levels, markets, company valuations and more.
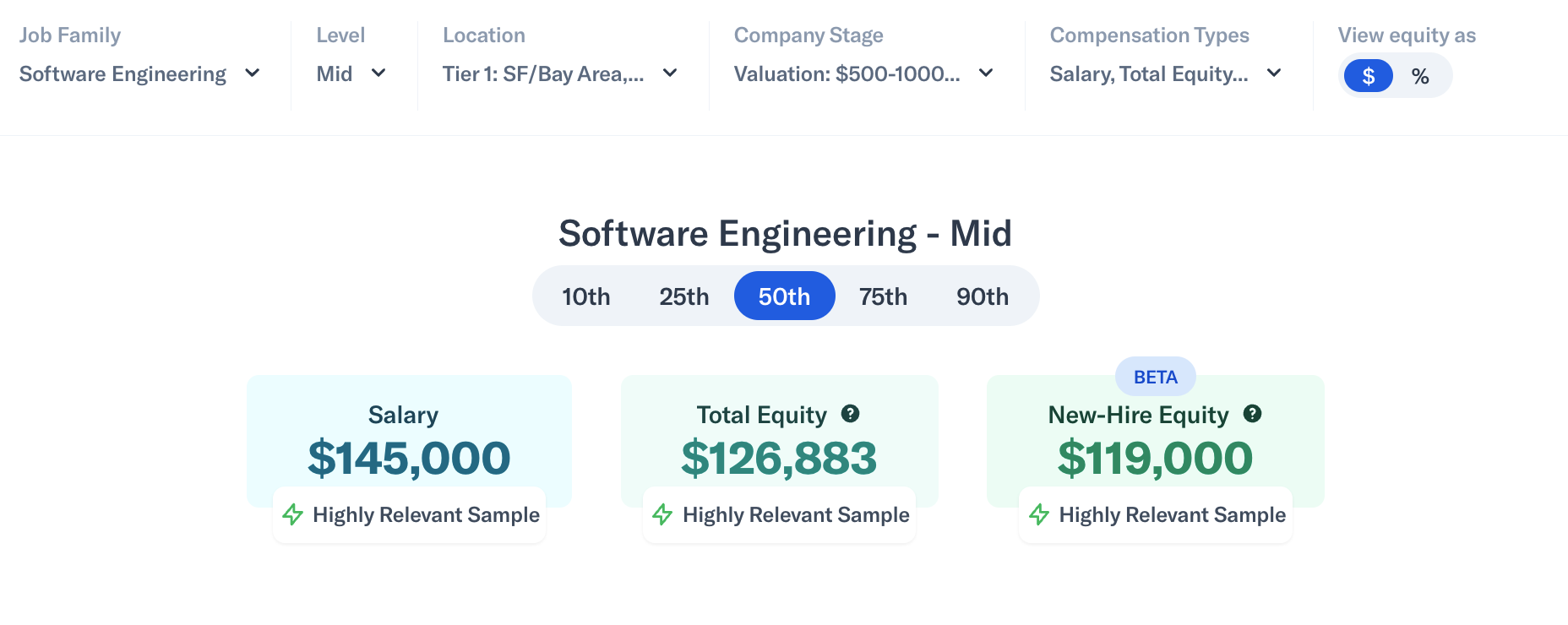
New Hire Equity helps teams:
- Contextualize equity benchmarks: Understand the ‘how’ behind total equity benchmarks, tracking how valuation growth and refresh shares contribute to employee equity packages.
- Make faster decisions more confidently: Access real-time new user equity data to make better equity-based comp decisions.
- Improve offer acceptance rate: Deliver more compelling and competitive offers to candidates that are backed by real-time data.
- Delight prospects: Enable companies to share rationale behind the equity grants they’re offering, which makes candidates feel more confident that they’re being compensated fairly.
- Stay ahead of the market: Win candidates with the right mix of compensation that stays up to date.
Ultimately, Pave exists for one reason: To make compensation fair. And compensation can’t be fair if people don’t have access to good data.
One of our customers, Julie Menge, Senior People Operations Managers at Mux, sums up the value of having reliable benchmarking data well:
"Equity is a key part of how we compensate at Mux. Creating competitive packages is core to our compensation philosophy, so it’s crucial to have reliable, relevant market data. With Pave, we're confident that we’re not only making fair offers, but also ensuring that our candidates completely understand the equity component of the total compensation we’re offering."
How to Get Started
Starting today, our customers can start using this product feature to improve their hiring outcomes, reduce bias in the offer process, and pave a path towards transparency in compensation decisions
We felt personally compelled to launch this feature to solve a problem we felt internally, and we hope it serves you well!
To start using New Hire Equity, simply navigate to Pave’s Benchmarking product and select the New Hire Equity option when setting your search criteria. If you’re new to Pave, you can sign up for free here!
If you want to learn more about how to use equity to improve acquisition and retention, view our on-demand equity webinar.
Nitin is the head of the Pave Benchmarking team.